Chatbot Chernobyl
The public release of ChatGPT has permanently contaminated the Web. Welcome to the world of Average Garbage Forever.
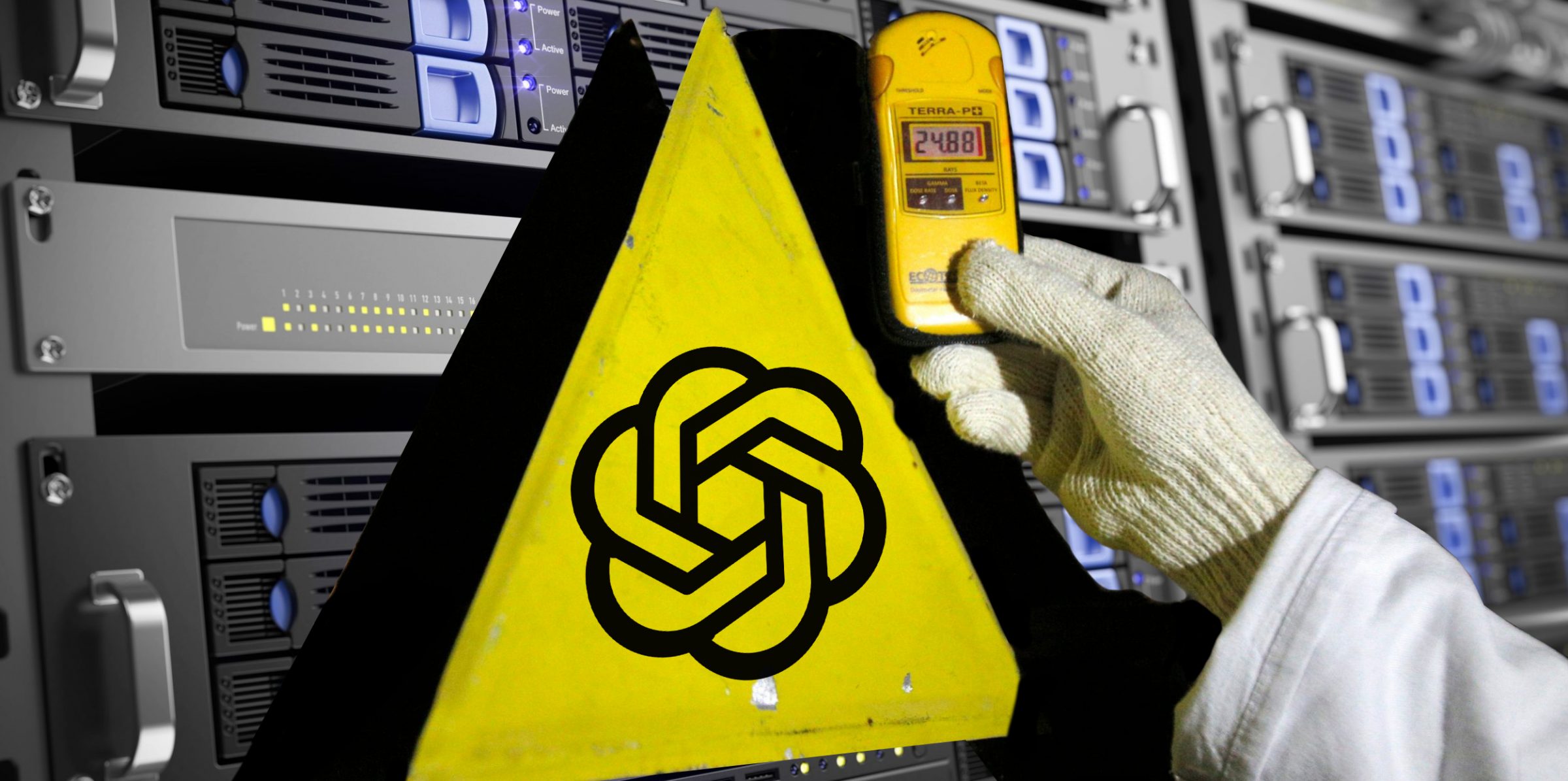
During the project to develop the first atomic bomb some of physicists involved briefly entertained the possibility that a fission explosion might generate enough heat to trigger a runaway chain reaction that would ignite the atmosphere and turn our planet into a second sun. Lengthy calculations provided some reassurance but, on the eve of the first test detonation – designated ‘Trinity’ – the remote possibility of armageddon still lingered in the minds of some attendees. In an attempt to relieve the tension, Italian physicists Enrico Fermi took bets from his colleagues on whether the explosion would wipe out all life on earth.
Thankfully, Trinity adhered to the mathematical predictions. The explosion left a crater eighty meters wide and turned a vast stretch of the surrounding desert into glass but there was no nuclear chain reaction. Nevertheless the test did have one unforeseen, world-altering consequence – it made it harder for humanity to work with radioactive material.
This is because the test – and the dozens of others that followed – scattered trace amounts of radioactive dust into our atmosphere and, while this fallout posed very little danger to living creatures, it found its way into all newly manufactured steel. Owing to the enormous volume of oxygen required to power industrial blast furnaces, every ounce of steel produced after 1945 retained traces of this background radiation – making it difficult to manufacture certain scientific and medical devices. It turns out that it’s difficult to build instruments that measure radiation using materials that are, themselves, radioactive.
So what does this have to do with the ‘AI’ chatbots that have been released to the public in recent months?
Well, much like the Trinity test, the recent release of ChatGPT has irrevocably altered the atmosphere of the Web. As of the 30th of November, 2022, OpenAI’s chatbot has been steadily producing vast quantities of ‘generative text’ in response to user prompts. This material can’t be detected and it can’t be recalled. From now on, everything we read and interact with online will be ever so slightly tainted by the suspicion of having been written by an application with no ability to gauge the accuracy of its pronouncements and no coherent understanding of the world. ChatGPT’s imitators will only add to this growing form of contamination.
No doubt this might sound a little hyperbolic. After all, the atmosphere of the Web has never been particularly pure – ever since the early 2000s most web traffic has consisted of unsolicited emails, pornography, banner ads, SEO-driven blogspam and a vast tailing pond of recycled memes, clickbait and listicles. But even if the current internet is 95% garbage, ChatGPT and other so-called ‘AI’ tools have the potential to compound this sad state of affairs by thoroughly crowding out the last remnants of human expression.
But what is ChatGPT?
Before getting into the pollution problem it’s important to understand what ChatGPT is and how it works. OpenAI’s ChatGPT (which stands for Generative Pre-trained Transformer) belongs to a category of software applications known as language models (LM) which are designed to calculate the probability of a particular sequence of words. Historically, language models have been used to assist with speech recognition and machine translation software and to improve search algorithms. Now they’re being pitched as a sort of jack-of-all trades AI assistant. You ask a question and ChatGPT spits out an answer in the form of ‘generative text’.
The latest version of OpenAI’s language model – GPT-3 – is trained on a dizzying 45 terabytes of digitised text. This database includes English language news archives, forum posts, blog articles, digitised books and the back catalogue of every major scientific journal. To give some idea of the scale of GPT’s training data – the entirety of Wikipedia (all 21 gigabytes of text) constitutes just 0.05 per cent of the total.
In order to identify patterns in this immense volume of information, AI researchers rely on ‘neural networks’ – mathematical systems which are modelled on the web of neurons in the mammalian brain. But any resemblance between digital neural networks and their biological equivalents is superficial at best. For the most part these applications reduce complex information to simple patterns and, because they have no way of evaluating the accuracy of the material they’re trained on, this process tends to amplify and enshrine social biases.
This is a real problem given the sort of texts that ChatGPT is trained on. Because alongside a lot of relatively reputable sources of information, ChatGPT’s training data includes an ocean of unfiltered garbage.
In a move that should reassure no one, OpenAI has indirectly outsourced the curation of this bilge to the users of Reddit. One of the key archives that GPT relies on – WebText2 – is comprised of text from every website posted to Reddit that managed to get more than three upvotes. This terrifyingly low bar ensures that ChatGPT’s training data includes the collected works of the internet’s most prolific/autistic posters. It means that, alongside essays by Hannah Arendt and Bertrand Russell, the application has likely absorbed a five page debate on bodybuilding.com about how many days there are in a week and the grand unified theory of Timecube. Thanks to open-source aggregators like Common Crawl, every unhinged rant, conspiracy theory and forum flame war that’s ever been punched into a keyboard is now available as source of AI inspiration.
To be fair to OpenAI, describing their training data as ‘unfiltered’ is a slight exaggeration. As mentioned in a previous post on Potemkin AI, any claims of ‘artificial intelligence’, ‘robots’ or ‘automation’ should prompt us to look for the hidden human labor behind these technologies. In the case of ChatGPT this workforce can be roughly divided into ‘data cleaners’ and ‘bot trainers’.
By any reasonable standard OpenAI’s data cleaners have one of the worst jobs imaginable. Their task is to help build automated content moderation tools by screening and labelling the most toxic material embedded within GPT’s training data. A recent investigative report by Time magazine described what this entailed:
“To get those labels, OpenAI sent tens of thousands of snippets of text to an outsourcing firm in Kenya, beginning in November 2021. Much of that text appeared to have been pulled from the darkest recesses of the internet. Some of it described situations in graphic detail like child sexual abuse, bestiality, murder, suicide, torture, self harm, and incest.”
By attempting to sanitise these subjects OpenAI hoped to avoid the sort of backlash that accompanied the release of Microsoft’s Tay chatbot back in 2016 whose users quickly trained it to parrot Nazi talking points.
The second group of ‘ghost workers’ that make ChatGPT possible are the trainers. These people are employed to ‘fine-tune’ the language model by running thousands of prompts through the bot and ranking the responses according to their relevance, Trainers are also expected to painstakingly enter in ‘desired responses’ whenever the bot comes up short. When Microsoft released Tay into the wild this task was left to the general public – the most dedicated of whom managed to convert the bot into a fountain of hate speech. OpenAI appears to have learned from this mistake by conducting the first phase of this fine-tuning process themselves but the public release of ChatGPT is, in many respects, a continuation of this training process – as every response is accompanied by thumbs up/down buttons that allow users to provide their own feedback.
In a final attempt to impose guardrails on their application OpenAI also blocked certain keywords and phrases but users quickly discovered that these restrictions were hilariously easy to circumvent. Ask ChatGPT how to hot-wire a car and it’ll respond by saying that doing so would be ‘against [its] programming’, but if you ask it to adopt the persona of a car thief, or recite hot-wiring instructions in the form of a poem, the application will happily comply.
What does ChatGPT allow you to do?
Most of us have already been exposed to a more limited version of ChatGPT’s underlying technology thanks to Gmail’s ’Smart Compose’ feature. When you draft an email with a common phrase Gmail will offer up the typical conclusion to your sentence. Theoretically, the more emails you write the better it gets at anticipating your particular turn of phrase. But, again, it’s worth remembering that ‘Smart Compose’ is not smart in any meaningful sense of the world. The application is simply recognising patterns and suggesting shortcuts.
But if ‘Smart Compose’ is just predictive text for sentences, then ChatGPT is just predictive text for entire documents. String together enough cliches in gmail and you get roughly the same effect. This is not to diminish the mathematical achievement that makes this possible or to deny the sometimes uncanny results. In certain circumstances these bots can produce responses which are genuinely impressive. Given only a brief prompt, ChatGPT can spit out several paragraphs of seemingly coherent text and if you ask it to revise its answer based on a new set of criteria you can drill down toward something halfway interesting.
Left to its own devices, however, ChatGPT produces bland, homogenised corporate prose. Because language models can only rephrase the data they’ve been trained on, they excel at producing ‘new’ versions of standardised documents. Among other things, ChatGPT can generate very convincing corporate press releases, boilerplate terms and conditions agreements, breaking news articles, real estate listings, product reviews and high school English essays.
The real novelty, however, comes from asking the bot to mimic a specific style of prose. For instance, when asked to describe losing a sock in the dryer in the style of the U.S. Declaration of Independence, ChatGPT produces a suitably ponderous response:
“When in the course of household events, it becomes necessary for one to dissolve the bonds that have connected a sock to its mate, and to assume among the powers of the laundry room, the separate and equal station to which the laws of physics and of household maintenance entitle it, a decent respect to the opinions of socks requires that it should declare the causes which impel it to go missing.
We hold these truths to be self-evident, that all socks are created equal, and are endowed by their manufacturer with certain unalienable rights…
When asked to describe a trip through a McDonald’s drive-thru in the style of Tim Winton it manages to crowbar an ocean metaphor into each paragraph.
As I pull up to the McDonald’s drive-thru, the sea air rushes past my face, reminding me of the rugged beauty of the western coast. I can hear the sound of the waves crashing against the shore, a constant reminder of the raw power of the ocean.
I press the button for the speaker, and the voice of the cashier crackles through the static. I order a burger and fries, a simple meal in this complicated world. I can feel the anticipation building within me, like the swell of a coming storm. . .
When asked to produce a tweet about James Cameron’s Avatar in the style of Slovenian philosopher Slavoj Zizek it produces something that sounds relatively Zizekian:
“The spectacle of #Avatar is not just a visual marvel, it reveals the fetishistic logic of late capitalism: we are all trapped in a blue body, yearning for a return to a pre-industrial Eden that never truly existed. #SlavojZizek #Cinema”
But each of these examples reveals the shortcomings of the model. The Declaration of Lost Socks doesn’t actually make sense, McWinton can’t tell if he’s at the beach or just remembering the beach and the Zizek Bot assumes that the humans on Pandora were trapped in their blue avatars. It’s no surprise that ChatGPT produces these nonsensical answers. It doesn’t actually understand what it’s being asked to do – it can only generate a probabilistic grab-bag of the words and phrases associated with the prompt.
That being said, it’s genuinely hard to write about language models and chatbots without implying that these programs ‘know’ or ‘understand’ your intentions in some way. It doesn’t help that researchers refer to ‘neural networks’, ‘emergent behaviour’ and ‘hallucinations’, or that journalists tend to adopt the widest possible definition of ‘Artificial Intelligence’.
In a recent article for the The New Yorker, science fiction author Ted Chiang likened ChatGPT to a vast, low-resolution JPG of every piece of writing that has ever been published online. However, in their efforts to compress all that data, OpenAI has discarded much of the fine-grain detail – leaving a snapshot that only resembles the original text. But as Chiang points out, we’re primed to interpret this blurriness as intelligence.
“The fact that ChatGPT rephrases material from the Web instead of quoting it word for word makes it seem like a student expressing ideas in her own words, rather than simply regurgitating what she’s read; it creates the illusion that ChatGPT understands the material. In human students, rote memorization isn’t an indicator of genuine learning, so ChatGPT’s inability to produce exact quotes from Web pages is precisely what makes us think that it has learned something. When we’re dealing with sequences of words, lossy compression looks smarter than lossless compression.”
And this appearance of intelligence is what makes language models like ChatGPT so seductive and, ultimately, so dangerous. Because they look intelligent it’s inevitable that they’ll be given responsibilities beyond what anyone should expect of a glorified Magic 8 Ball. As it happens, individuals and organisations are already using chatbots to make decisions, field inquiries and inform the public. Microsoft’s long-marginalised Bing search engine has recently been upgraded to incorporate LLMs and Google is set to follow with their own AI-assisted search functionality.
But the first real detour into Black Mirror territory occurred in early January when the CEO of the online mental health service Koko announced that his company had used GPT 3 to offer mental health advice to ‘about 4,000 people’. In a twitter thread that went viral for all the wrong reasons the company’s CEO, Rob Morris, proudly announced that Koko had incorporated the model’s responses into online counselling sessions for suicidal teenagers. Morris said that this approach helped reduce response times but admitted that any therapeutic effects disappeared when clients discovered that they were being fed automated replies. “Once people learned the messages were co-created by a machine, it didn’t work” he wrote “Simulated empathy feels weird, empty”.
As well as being blindsided by the obvious, Morris appears to have been unaware of the legal and ethical lines that his experiment crossed. Thankfully those who replied were quick to set him straight. ‘If I were in your study I would feel manipulated and used and compromised’ one person replied. ‘You deserve to be sued out of existence’ wrote another. One doctor who identified themselves as a former chair of a federal research review board informed Morris that he had effectively ‘…conducted human subject research on a vulnerable population without IRB approval’.
Despite the obvious limitations of language models, the public release of ChatGPT has been accompanied by a deluge of breathless commentary predicting a chatbot revolution that will erase jobs and upend entire industries. On social media, this hype was amplified by ChatGPT’s early adopters – software developers who used the prototype to generate and parse blocks of code (in addition to English, ChatGPT’s training data included large volumes of Python, C, Javascript and other coding languages and frameworks). For this type of task ChatGPT performed surprisingly well – parsing and producing workable code (even if it wasn’t always the code that the user had asked for) and providing line-by-line descriptions of each function.
At the same time, it was also becoming apparent that academic fraud would be one of the main use cases for next-gen chatbots like ChatGPT. In an effort to get a grip on this new spanner in the works, representatives of some of the softer sciences began to test out ChatGPT for themselves. But when faced with typical English or history essay prompts, the results were far less impressive.
Teachers noted that the ChatGPT tended to produce vague and circular arguments, that it made routine factual errors and that it occasionally ‘hallucinated’ entirely new events which it nonetheless backed up with fake references. Further testing revealed that ChatGPT fabricates its responses roughly 20% of the time but these hallucinatory answers – some painfully obvious – are always phrased as certainties1.
The falsification of references is disconcerting but it’s also revealing of the way language models like ChatGPT work. In these instances the bot only ‘knows’ the rough shape and format of a scientific paper. It recognises that essays have paragraphs and that some of the sentences within those paragraphs are followed by surnames and dates in parentheses. Thus it reproduces those elements based on probabilities without any meaningful understanding of what they signify. The end result looks like an audacious attempt at academic fraud2.
Because they have no way of storing definitions or making connections between concepts, language models cannot make inferences or produce novel arguments. At best, they can regurgitate mangled versions of the opinions found in their training data but this process inevitably introduces errors. Historian Bret Devereaux provided this example in his summary of the academic impact of ChatGPT:
“To put it one way, ChatGPT does not and cannot know that “World War I started in 1914.” What it does know is that “World War I” “1914” and “start” (and its synonyms) tend to appear together in its training material, so when you ask, “when did WWI start?” it can give that answer. But it can also give absolutely nonsensical or blatantly wrong answers with exactly the same kind of confidence because the language model has no space for knowledge as we understand it”
Despite these deficiencies, people have still managed to use chatbot responses to achieve passing grades in certain postgraduate courses. One recent experiment at the University of Pennsylvania business school demonstrated that GPT-3 could pass the final exam for its Master of Business Administration course. Another study – conducted using an earlier version of GPT – determined that bot-generated essays would have netted a C average across four undergraduate university subjects (in this case the bot’s marks were dragged down by an F in creative writing). As historian Ted McCormick noted in a recent twitter thread:
“The fact that ChatGPT writes strange and mediocre undergraduate humanities papers but fantastic elite MBA exams suggests it may not have the same implications for every kind of education”
The aforementioned experiments were conducted ‘blind’ – that is to say the reviewers were not told that they were assessing bot-generated essays. But even staff who have been trained to recognise generative text can’t consistently distinguish between essays generated by bots and those submitted by disinterested students. Moreover, the software currently used to detect plagiarism has no way of identifying the output of chatbots – which produce original (if somewhat derivative) answers.
Some commentators have suggested that specialised software designed to detect generative text will provide a solution to this impasse but the current crop of ‘classifiers’ doesn’t offer much reassurance. Responding to pressure from academic institutions, OpenAI began work on their own detection tool soon after ChatGPT’s release but, in a statement on their website, they were quick to set low expectations.
“Our classifier is not fully reliable. In our evaluations on a “challenge set” of English texts, our classifier correctly identifies 26% of AI-written text (true positives) as “likely AI-written,” while incorrectly labeling human-written text as AI-written 9% of the time (false positives).”
Even if these literary Turing Tests improve over time, any arms race between bots and bot-detectors will inevitably result in innocent humans being caught in the crossfire. After all, how is a student supposed to prove that they didn’t ask Siri to do their homework?
Open AI’s CEO, Sam Altman, has shown very little sympathy towards educators who are now faced with the prospect of overhauling their approach to assessments in order to mitigate academic fraud. In an interview with StrictlyVC Altman said that:
“We’re just in a new world now. Generative text is something we all need to adapt to, and that’s fine. We adapted to calculators and changed what we tested for in math class, I imagine. This is a more extreme version of that, no doubt, but also the benefits of it are more extreme as well.”
Many of the cheerleaders for the AI revolution have welcomed the disruptive impact of chatbots on education – suggesting that they will reduce the emphasis on standardised testing and promote greater critical thinking. These proponents argue that, given the successful automation of essays, educational institutions should switch to teaching students how to formulate prompts to make the best use of the next generation of language models. Their underlying assumption seems to be that the purpose of academic essay-writing is to create essays in much the same way that the purpose of basket-weaving is to create baskets.
Needless to say, this is a pretty basic misunderstanding of what essays are for. At the risk of stating the obvious, students are asked to write essays so that they can learn how to conduct research, construct arguments and organise their thoughts. The document itself is simply evidence of those skills. The fact that no one actually wants to read a seventeen year old’s take on the French Revolution doesn’t mean that their efforts to write about the subject were wasted.
Proponents of this technology insist that GPT-3 and its imitators are only the beginning. OpenAI promises that the next iteration of their system will be more efficient and better able to mimic human behaviour but it’s not clear how greater fluency can overcome the basic limitations of a language model. Many overexcited commentators have jumped to the conclusion that the latest crop of chatbots are a stepping stone to a more generalised artificial intelligence but it’s far from clear that this is the case. It doesn’t matter how much additional training data gets fed into a language model, it’s not going to suddenly achieve sentience. As historian Bret Devereaux was quick to point out, ChatGPT needs to be understood as an advanced version of predictive text rather than a ‘primitive version of Skynet’.
“I can imagine more tailored chatbots still being useful in producing some things – highly formulaic documents, for instance. But making a machine that can analyze, understand its material, and output an idea from that understanding – ChatGPT doesn’t even attempt that.
So many of the defenders of this technology see a carriage rolling downhill and declare that the creators have invented the automobile.”
The Real Problem
The difficulty of detecting generative text highlights the real problem with AI chatbots – data pollution. Here we return to the original radiation metaphor because, aside from cheating on written assignments, the main industry that will benefit from high-powered chatbots is the one that no one really wants to exist in the first place – content marketing. This is the technical term for all the low-quality articles, newsletters and how-to guides that clutter up Google’s search results. These pages are largely written for the benefit of search engines and they make money by occupying those high-traffic areas and selling advertising space.
This form of ‘search engine optimisation’ is why the internet is filled with long-winded articles which don’t actually answer the question you want answered. It’s why online recipes are often preceded by long, discursive explorations of tomato varieties or the author’s family history. More generally, it’s why Google’s search results keep steering you back to the same monolithic content mills.
Using GPT, sites like Mashable and CNET can produce an unlimited amount of this marketing spam without having to fund a small army of depressed freelance copywriters. Over the last decade the ‘handmade’ version of this content has displaced genuinely useful sources of information and contributed to the phenomena that tech journalist Cory Doctorow refers to as the ‘enshittification’ of the Web.
New Yorker columnist Kyle Chayker has also charted the devolution of Google’s search results from valuable commentary on discussion boards and personal blogs to cookie-cutter responses hosted on sprawling SEO-optimised corporate websites. In a recent twitter thread Chayker described the new paradigm as ‘Average Garbage Forever’:
“I’ll say again what I will doubtless be saying a million times in the coming years: Algorithmic feeds have pushed content creators to conform to the acceptable aesthetic and cultural average; A.I. generation will just automatically produce that average from the start”
A preview of this brave new world was provided by journalist Jon Christian in a recent article for Futurism which revealed that CNET has already begun using language models to write articles for the site’s personal finance section – an interesting choice given that ChatGPT has proved to be notoriously bad at basic math. Over the course of their investigation staff at Futurism discovered numerous instances in which bot-generated articles misrepresented basic financial concepts or omitted crucial context from their explanations. Christian ultimately concluded that, while language models were good at ‘spitting out glib, true-sounding prose’, they appeared to have a ‘difficult time distinguishing fact from fiction’.
CNET responded to this expose with corrections and disclaimers indicating that certain articles had been ‘assisted by an AI engine’ but, in a follow up article, Christian raised the obvious question:
“If these are the sorts of blunders that slip through during that period of peak scrutiny, what should we expect when there aren’t so many eyes on the AI’s work? And what about when copycats see that CNET is getting away with the practice and start filling the web with their own AI-generated content, with even fewer scruples?
Obviously this problem is not confined to mediocre corporate marketing material. As covered in a previous essay, content marketing and political propaganda represent two sides of the same coin. As researchers Dipayan Ghosh and Ben Scott described in a 2018 whitepaper on fraud and deception online:
“Political disinformation succeeds because it follows the structural logic, benefits from the products and perfects the strategies of the broader digital advertising market”
Thus, the widespread availability of chatbots is destined to make life harder for all of us when it comes to dealing with online harassment and campaigns of political interference. Up until recently most bot spam was crude and relatively conspicuous. If you saw someone on Twitter with the default egg avatar post a bad take on some controversial topic you could copy their tweet into the search bar and discover the exact same message on dozens of other suspicious-looking accounts. However the current crop of ‘generative AIs’ will allow the same malicious actors to personalise their dummy accounts with unique headshots of people that don’t exist and churn out infinite variations of the same talking points – creating the illusion of popular support for fringe political beliefs.
Disinformation is already overwhelming more reputable sources of information. Anyone who’s argued with a vaccine sceptic or climate denier will have some appreciation for Brandolini’s law (AKA the bullshit asymmetry principle) which states that “the amount of energy needed to refute bullshit is an order of magnitude bigger than that needed to produce it.” With the public release of ChatGPT, OpenAI has reduced the cost of creating new bullshit to almost nothing. And for all the hype surrounding these new application, there’s no way to use bots to combat misinformation because language models have no concept of ‘truth’ and no way of assessing the validity of a given claim.
Conclusion
After Trinity contaminated our atmosphere, vintage metal became much more valuable. The demand for ‘low background’ steel saw salvage companies combing the ocean floor for shipwrecks that could be cut up and recycled for medical and scientific use. Germany’s WWI naval fleet – scuttled in shallow waters off Scotland in 1919 – became a valuable repository for untainted metal. Some 2,000 tonnes of steel from this windswept stretch of coastline were used to build medical devices (and their radiation-shielded enclosures) in hospitals all over the world. Ancient trade goods also received a second lease on life in scientific laboratories. In 2010 the National Archaeological Museum in Sardinia donated four tonnes of lead recovered from an ancient Roman shipwreck to the country’s national particle physics laboratory at Gran Sasso.
The good news is that background radiation is gradually becoming less of a problem. The half-life of Cobalt-60 is short (only 5.26 years) and a moratorium on atmospheric testing in 1960s has successfully reduced the level of atmospheric radiation (despite the occasional spike from meltdowns like Chernobyl and Fukushima). Thanks to software that corrects for radioactive interference, the necessity of finding new sources of ‘low background’ steel has also diminished.
It’s unlikely that we’ll see such a happy outcome when it comes to generative text. Given the growing volume of generative images, audio and text, a better metaphor for the release of ChatGPT might be the meltdown that occurred at the Chernobyl nuclear power plant in 1986 or the oil spill that resulted from the sinking of the Deepwater Horizon in 2010. In both these cases the authorities eventually managed to seal off deadly leaks but, when it comes to generative AI, the rupture is widening by the day.
As of January this year OpenAI laid claim to about 100 million registered users. Rough calculations suggest that their application could be spitting out 300 million words per minute3 and while most of this material is probably being viewed and discarded, some unknown fraction is undoubtedly finding its way onto the Web in the form of blogspam, disinformation campaigns, marketing guff and last-minute homework assignments. As competing ‘AI’ platforms come online, generative text will become an unavoidable blight on the internet – displacing human insight and watering down useful information in every domain.
In his ‘Blurry JPEG’ article for The New Yorker, Ted Chiang predicted that, when it’s released, GPT-4 will probably attempt to ignore text generated by GPT-3. If this turns out to be the case it will be confirmation that OpenAI doesn’t consider the output of its own language model to be a reliable source of information. It’s not clear what a chatbot trained on generative text would produce but it’s unlikely to be anything worth reading. In the words of Cory Doctorow:
“…feeding a new model on the exhaust stream of the last one [will] produce an ever-worsening gyre of tightly spiraling nonsense that eventually disappears up its own asshole.”
Given their current inability to identify their own handiwork, it’s far from certain that OpenAI will be able to filter out generative text. Instead they may have to continue limiting their training data to material published before 20224. One possible outcome of the current chatbot gold rush is that future generations may forever be stuck searching for certainty in the entrails of the early Web.
Footnotes
(1) This tendency to produce nonsense is not confined to language models. When prompted to depict humans, AI image-generators like Midjourney are capable of producing photorealistic images of people that, on closer examination, turn out to be Cronenburg-esque mutants with additional hands, knots of fingers and excess teeth. This is because generative art bots don’t possess a fundamental knowledge of geometry or physiology – instead they can only recognise patterns and guess at how many fingers a hand might have or what they would look like when viewed from a certain angle. By the same token, language models lack any baseline knowledge of the subjects they’re prompted with – hence they produce a lot of meandering and repetitive prose.
(2) Fabricated citations are especially annoying because verifying them can take a substantial amount of time. In the AIED experiment the GPT-generated essay cited a real journal and real authors (who’s work would have been relevant to the discussion) but it fabricated the issue number of the journal and the publication date.
(3) By comparison, Twitter’s entire user-base generates roughly 2.8 million words per minute.
(4) For the moment languages other than English have been spared this contamination but, given the prevalence of automated translation tools, generative text is certainly going to break out of its loose quarantine in the English Web.
References:
Jon Christian (2023) – Leaked Messages Show How CNET’s Parent Company Really Sees AI-Generated Content
Mike Sharples (2022) – Automated Essay Writing: An AIED Opinion
BestUniversities.net (2023) – What Grades Can AI Get in College?
Emily M. Bender et. Al. (2021) – On the Dangers of Stochastic Parrots: Can Language Models Be Too Big?
Cade Metz (2020) – Meet GPT-3. It Has Learned To Code (and Blog and Argue)
Brian Hayes (2015) – Crawling toward a Wiser Web
OpenAI Blog (2023) – New AI classifier for indicating AI-written text
Dmitri Brereton (2022) – Google Search Is Dying
Cory Doctorow (2023) – The ‘Enshittification’ of TikTok
Connie Loizos (2023) – Strictly VC in conversation with Sam Altman
Robert Aizi (2022) – Testing Ways to Bypass ChatGPT’s Safety Features
Jill Rettburg (2022) – ChatGPT is multilingual but monocultural, and it’s learning your values
Timnit Gebru et al. (2021) – Datasheets for Datasets
Billy Perigo (2023) – Exclusive: OpenAI Used Kenyan Workers on Less Than $2 Per Hour to Make ChatGPT Less Toxic
Life Architect (2023) – Inside language models (from GPT-3 to PaLM)
Kindra Cooper (2021) – OpenAI GPT-3: Everything You Need to Know
Stephan Wolfram (2023) – What Is ChatGPT Doing … and Why Does It Work?
Viki Auslender (2021) – Meaningless words: Dangerous conversations with ChatGPT
Jed Oelbaum (2018) – The Worldwide Scavenger Hunt For Vintage, Low-Radiation Metals
Leave a Reply